F5.5G Leap-forward Development of Broadband in Africa The Africa Broadband Forum 2024 (BBAF 2024) was successfully held in Cape Town, South Africa recently, under…
Face to face with facial recognition: the basics, the uses, the myths
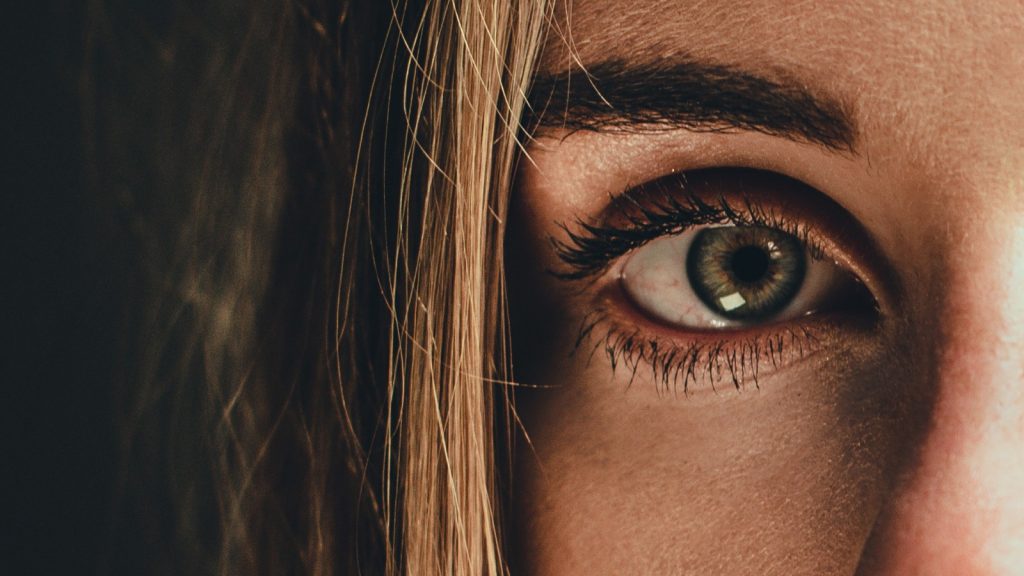
The internet loves buzz words: AI, machine learning, biotechnology, natural language processing, neural networks, gene editing, robotic process automation, and collective intelligence with few the wiser on what these terms actually mean or how it will impact their lives.
If your job title is Chief Robot Whisperer or Hacker in Residence, you may have some idea, but for most the advances in technology either confound and scare them or confound and excite them.
A technology I am particularly excited about is that of facial recognition and analysis.
Powered by artificial intelligence and more specifically machine learning, the technology has existed for decades.
As computing power and machine learning algorithms have improved, so too has facial recognition and the ways in which it can be applied.
The basics
Facial recognition is a system built to identify a person from an image or video.
To be more precise, recognition software identifies unique facial and body features and translates them into a unique ID, which is then used to link the unique features to a user and or the user’s information.
Although the first experiments with semi-automated computer-based facial recognition were made during the mid-1960s by Woodrow Wilson Bledsoe, it has only been in the last few years that the technology has become more ubiquitous.
In all likelihood, you are already using the tech without even realising it.
For example, if you use your face to unlock your phone or personal photo apps to automatically identify people who regularly appear in your pics, then “tag you’re it”.
How it works
Facial analysis capabilities allow users to understand where faces exist in an image or video, as well as the attributes of those faces.
For example, the software analyzes attributes such as how far apart your eyes are, what colour they are, what your mood is, what colour your hair is and what the visual geometry of your face is.
The tools work by offering a confidence score that they are correct in their assumption. In other words, they predict how accurate they think their assumption is.
The machine learning algorithms are trained on datasets of hundreds of thousands of images and continuously improve as more data is added and more faces are analysed.
Misconceptions
Understandably there has been a lot of uneasiness and apprehension around how the technology is being and could be used in the future. To this end, it is important to dispel some common misconceptions.
First, despite what you may think, machines are better at recognising faces than humans are.
The National Institute for Standards and Technology (NIST) recently shared a study of facial recognition technologies that concluded that even older technologies could outperform human facial recognition capabilities. Hard to believe, but true.
Although we expect all humans to be biased to some degree, one of the biggest concerns and push back for the use of facial recognition technology is the potential that the technology itself is biased when it comes to recognising age, race or even gender and that actions will be taken, or not taken, based on the algorithms’ result.
This inherent bias may be as a direct result of the data on which the algorithms are being trained.
For facial recognition technology to perform as desired — to be both accurate and fair– training data must provide sufficient balance and coverage.
The training data sets should be large enough and diverse enough to learn the many ways in which faces the world over differ. In other words, the images must reflect the diversity of features in faces globally.
It’s therefore crucial that the creators and implementors of this technology both plan and audit for representation in their data.
Fortunately, there are some fantastic initiatives under way such as the million-image Diversity in Faces (DiF) set which IMB is creating to ensure large and diverse enough data sets are in fact available for creators. As with any AI system, the algorithm is only as good as the data on which it is built and trained.
Second, as in all probabilistic systems, the mere existence of false positives does not mean that facial recognition is flawed. Rather, it emphasises the need to follow best practice, such as setting a reasonable confidence score threshold that correlates with the given use case.
For example, Amazon Web Services, the creators of AWS Rekognition, advises that when facial recognition technology is used by law enforcement for identification, or in a way that could threaten civil liberties, a 99% confidence score threshold should be applied.
So depending on how and why the technology is being used, the confidence score should be set accordingly. Think of the confidence scores as a measure of how much trust a facial recognition system places in its own results; the higher the confidence score, the more the results can be trusted.
One of the major advantages of this technology is that it continuously learns and improves and therefore false positives can and will be reduced over time.
Use cases
The use cases for facial recognition are endless. From finding missing children using the International Centre for Missing and Exploited Children’s (ICMEC) GMCNgine to checking in at your local gym to paying for your next cheeseburger by simply smiling at the camera, the ways in which the technology can be applied is growing in leaps and bounds.
Some of the latest and more creative examples include Master Card Identity Check and Alibaba’s Smile to Pay.
For those living in West Africa, Aella Credit provides instant loans to individuals with a verifiable source of income in emerging markets by using biometric and employer data. Even tourism destinations are utilising the tech.
In Wuzhen, China, visitors are now asked to upload a selfie to access key areas of the historic town. The technology has shortened wait times and added convenience for guests.
From art to mapping to influencer marketing to healthcare, recognition technology is being used in more and more ingenious ways.
Facing the future
New technology can be daunting, the unknown usually is, but it should not be banned or condemned because of its potential misuse.
Instead, there should be open, honest, and earnest dialogue among all parties involved to ensure that it is applied appropriately and is continuously enhanced.
For those using and actively building facial recognition tools, we are all responsible for applying context limitations to systems we develop to mitigate harm, and it goes without saying that everyone should be schooling themselves on the best practices.
Keeping an eye on what the market leaders are doing is essential. For example, Microsoft is planning to implement self-designed ethical principles for their facial recognition technology by the end of March 2018 and are urging governments to push ahead with matching regulation in the field.
Similarly, AWS is publishing articles and lobbying government on how to apply facial recognition responsibly. Basics such as not violating an individual’s rights including the right to privacy and written, visible notices at premises where video surveillance, including facial recognition, are in use should be a no brainer.
Feature image: Ivandrei Pretorius via Pexels